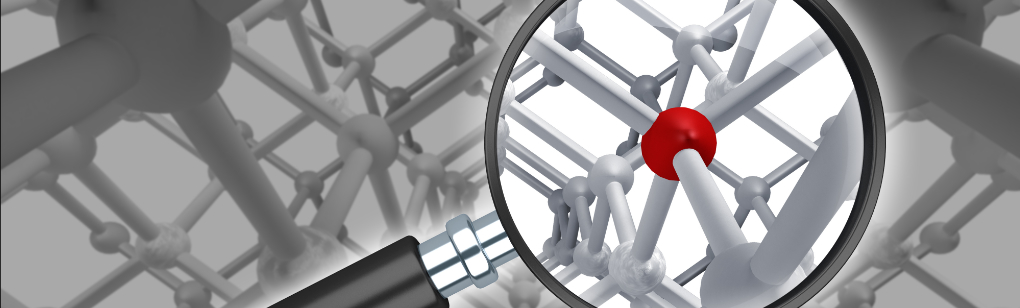
The business value of anomaly detection use cases in banks is obvious. From credit card fraud to check fraud to money laundering to cyber security, precise and quick anomaly detection is necessary to conduct business, protect customers and protect the bank from potential losses.
The best use case for machine learning-based anomaly detection in banking is fraud detection. With the US FTC having received millions of fraud-related complaints in the last five years; the size of the problem is quite overwhelming.
However, it is not easy to build an accurate system that works in real-time, as the number of fraudulent card charges is much lower than the total volume (Visa processes over 2,000 transactions every second) thus making establishing a training set difficult.
Conventional rules-based systems are inadequate, often resulting in false positive rates that exceed 90%. This creates a massive number of false positive alerts which then need to be cleared using manual intervention. Repetitive analyst action can lead to a “numbness to false positives,” raising operational risk and therefore regulatory risk.
Additionally, traditional systems are reactive, as they only generate alerts based on previous rules.
Alternatively, AI-based systems are proactive and can help strengthen regulatory alert systems and improve analysts’ workflow by reducing noise without dismissing alerts.
Gaining an understanding of what data science, machine learning, and AI can bring to fraud detection and other anomaly detection use cases in banking is a first step to making headway in these areas.
Combining this knowledge with more overarching AI project best practices, including operationalization and data democratization, helps banks stay ahead of the curve.
Let’s see the 5 key considerations when working with anomaly detection:
1. Selecting and understanding the use case
The first step in successful anomaly detection is to understand the kind of a system the line of business needs and to lay out a framework for the requirements and objectives. These are important preliminary discussions because not all anomaly or fraud detection work is the same; exactly what qualifies as an anomaly and the subsequent processes kicked off by anomaly detection vary vastly by (and even among) use cases.
The nature of the data, the problem at hand, and the project goals must decide the techniques used for anomaly detection.
Even within banks, different projects will have different definitions of what makes a data point an anomaly. For example, tiny fluctuations in a system tracking stock prices, could be considered anomalies, while other systems like card charge location could tolerate a much larger range of inputs.
So one cannot universally apply a single approach as for other types of data projects.
Fraud detection is a good use case for a proof-of-concept in a bank as it does not examine as many social factors as stock trading to make recommendations and does not attempt to drive transactions itself.
This is a useful “gateway model” to implement and increase familiarity with AI project processes. But for banks already integrating AI-driven systems, a more advanced quantitative stock trading use case might be an appropriate place to experiment with anomaly detection.
To ensure the success of an anomaly detection project, it is vital to bring together technical profiles carrying out the work (data scientists, quants, or actuaries) with the business side (risk team, analysts) to:
- Define and continually refine what constitutes an anomaly. The definition may constantly change, which means continual re-evaluation.
- Define the goals and parameters for the overall project. For example, the end goal is probably not just to detect anomalies, but something larger that impacts the business, like blocking fraudulent charges. Knowing the bigger picture enables better definition of the project scope and the expected outcome, and is critical to gain user and stakeholder buy-in.
- After an anomaly is detected, determine what the system will do next. For example, send the anomalies to another team for further analysis and review.
- Develop a plan to monitor and evaluate the success of the system.
- Define the ideal anomaly detection frequency (real-time vs. batch) for the use case.
2. Getting the data
Having as much data for anomaly detection as possible enables more accurate models because one never knows which feature might be indicative of an anomaly. Using multiple types and sources of data is what allows banks to go beyond point anomalies to identifying sophisticated, contextual or collective anomalies.
For example, it is possible that the transaction data is not anomalous because the fraudster has stayed within the “normal” range of the actual user’s behaviour. However, data from ATM use or account weblogs may reveal anomalies.
3. Exploring, cleaning, enriching the data
When performing anomaly detection, this stage is important, because often the data contains noise (usually errors, either human or otherwise) which tends to be similar to actual anomalies. So, it is critical to distinguish between the two and remove any problematic data that could produce false positives.
Ideally, there would be sufficient amount of labeled data from which to begin; that is, analysts or data scientists would be able to enrich the datasets from the bank with information on which records represent anomalies and which are normal.
If possible, starting with data known to be either anomalous or normal is a good way to begin building an anomaly detection system because it will be the simplest path forward, enabling supervised methods with classification (as opposed to unsupervised anomaly detection methods).
For some of the use cases mentioned earlier, this is quite attainable. In fraud detection cases there is a clear mechanism for feedback that creates anomalous cases (e.g. customer relationship manager data detailing fraud complaints).
4. Becoming predictive
There are two primary frameworks for building anomaly detection systems:
- Supervised anomaly detection, which can be used if there is a labeled dataset where we know whether each datapoint is normal or not.
- Unsupervised anomaly detection, where the dataset is unlabeled (i.e., whether or not each datapoint is an anomaly is unreliable or unknown).
When using a supervised approach, it helps to apply a binary classification algorithm. Exactly which algorithm is not as important as taking appropriate measures regarding class imbalance (i.e., the fact that for anomaly detection, it’s highly likely that there are much more “normal” cases than anomalous ones).
When using an unsupervised approach, there are two ways of training algorithms:
- Novelty detection: The training set is made exclusively of inliers so that the algorithm learns the concept of “normality” (hence the prefix “one-class” found in some methods). During testing, the data may also contain outliers. This is also referred to as semi-supervised detection.
- Outlier detection: The training set is already contaminated by outliers. The assumption is that the proportion of outliers is small, so that novelty detection algorithms can be used. Consequently, those algorithms are expected to be robust enough at training time to ignore the outliers and accommodate only the inliers.
Visualizations are useful when building and testing anomaly detection models because they are the clearest way to see outliers, especially in voluminous datasets.
5. Deploying and iterating
To maximize the impact of an anomaly detection system, the model should score data in real-time in production.
Anomaly detection in banks are extremely time-sensitive, so going to production to make predictions on live data instead of test or stale data is critical. This can be challenging with sensitive personal financial data, which is typically available to a limited number of trusted users and systems.
In all financial anomaly detection use cases, data governance and privacy are dilemmas, but they don’t have to come in the way of model implementation.
But deploying a model in production is not the end. Iteration and monitoring of fraud and any other anomaly detection systems is critical for ensuring that the model continues to learn and be agile enough to continue detecting anomalies even as environments and behaviors change.
However, unlike other types of machine learning models, accuracy is not a practical measure for anomaly detection.
Since the vast majority of data is not anomalous (there could be hundreds of thousands of “normal” data points), the system could achieve a very high accuracy but still not actually be accurately identifying anomalies.
It is evident that minimizing fraud with anomaly detection is important to users and stakeholders in banks, but the question is where to begin.
Beginning with concrete, incremental goals, for example a 5% increase in flagging fraudulent behavior, or a 10% increase in detection speed, helps articulate clear ROI and besides indicating future room for growth.
Also, by prioritizing integration into existing systems, rather than overriding workflows, ML-powered fraud detection is more likely to work within a particular bank, so that users and stakeholders can then gradually develop trust and cross-verify potential mistakes.
Integrating a complete fraud detection overhaul overnight is overambitious, but by selecting one use case and encouraging teams’ adoption, banks can provide enormous value and peace of mind to customers and shareholders.